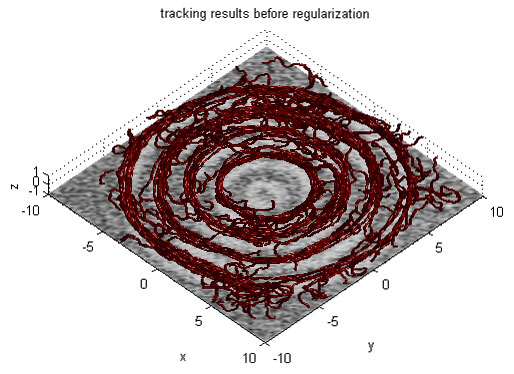
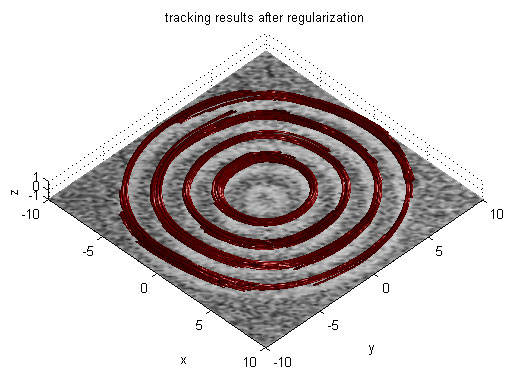
Magnetic Resonance Diffusion Tensor Imaging (MR-DTI) is a relatively new MR imaging technique which is based on measuring the extend of diffusing water molecules in 3D space. The technique is solely based on measuring the phase differences (via signal suppression due to phase differences) between spinning water molecules. This phase difference occurs due to the diffusion process because different molecules diffusing in different directions experience different magnetic fields (due to the spatially varying B-field). The output is a set of Diffusion Weighted Images (DWI). The diffusion tensor is mathematical structure that summarizes (upto second order accuracy) this diffusion process, i.e. the information in this set of diffusion weighted images. We suggest R. Bammer's, from Stanford University LUCAS MRS/I Center, PhD thesis as a complete reference for MR-DTI. A fundamental problem related to DTI is that you need to monitor (listen to the MR signal) a sufficiently large volume (voxel), after waiting for sufficiently long time to see the effect of anisotropic diffusion (if there is any). Furthermore DTI itself is only a 2nd order approximate modeling of the true diffusion process. These issues impose limitations of the speed and resolution of DTI. In other words, DTI can not and will not be able to image single fibers. Consequently, the interpretation of DTI data needs reliable modeling regarding the information it contains.
Boğaziçi University
Volumetric Analysis & Visualization Group
Electrical and Electronics Engineering Dept
34342 Bebek / Istanbul
Tel: +90-212-3596465 / 3596414 (Dept.)
Fax: +90-212-2872465
Email: acarbu@boun.edu.tr